A 150-year-old problem resolved using AI
New approach enables precise predictions of the phase transition
Combining concepts from statistical physics with machine learning, researchers at the University of Bayreuth have shown that highly accurate and efficient predictions can now be made as to whether a substance will be liquid or gaseous under given conditions. They report their findings in the journal Physical Review X.
Under certain conditions, water can exist as a liquid and a gas at the same time, for example in cloud formation: Depending on the temperature, water vapor in the air condenses into liquid droplets. The theory of phase separation explains why and how a liquid and its vapor can split into two separate phases - liquid and gas. Experimental observations by Thomas Andrews in the late nineteenth century identified the existence of the critical point and shortly afterwards Johannes Diderik van der Waals (Nobel Prize 1910) described phase separation using a simple theoretical model. Van der Waals' theory of phase separation is textbook material but is based upon crude approximations. It remains difficult to predict whether a substance will be liquid or gas under given conditions. Modern statistical theories, such as classical density functional theory, go much further but are also based on approximations that are difficult to control. Dr. Florian Sammüller and Prof. Dr. Matthias Schmidt from the Chair of Theoretical Physics II at the University of Bayreuth, together with the British physicist Prof. emeritus Robert Evans FRS, the founder of classical density functional theory, have developed a new approach that enables precise predictions of the phase transition. They achieved this by combining theoretical physics and a so-called neural network: a computer model consisting of artificial "nerve cells" that are connected to one another and process information.
In their study, the researchers combined the powerful theoretical description with the accuracy of computer-simulations. The input data of a neural network is linked to a "functional relationship" formulated by Evans in 1979, according to which all properties of a system are determined solely by the particle density. "Until now, functional relationships were reserved for modeling through physical intuition and working with pen and paper. Machine learning now makes it possible to overcome the associated limitations improving accuracy enormously. A wealth of assumptions that have only been suspected since van der Waals can be quantitatively investigated and, surprisingly, largely confirmed very clearly," says Schmidt.
The hybrid methodology used, combining machine learning and fluid theory, offers broad future application potential in the flexible modeling of the behavior of substances and the phenomena that occur in them, such as the wetting of substrates, capillary behavior in pores, or demixing phenomena. "Theoretical physics, specifically the statistical mechanics of fluids, offers a wealth of concrete tests in the form of rigorous equations that allow the quality of AI predictions to be assessed and ultimately controlled," adds Sammüller.
Original publication
Other news from the department science
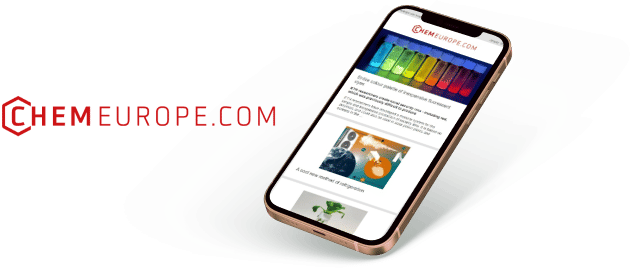
Get the chemical industry in your inbox
By submitting this form you agree that LUMITOS AG will send you the newsletter(s) selected above by email. Your data will not be passed on to third parties. Your data will be stored and processed in accordance with our data protection regulations. LUMITOS may contact you by email for the purpose of advertising or market and opinion surveys. You can revoke your consent at any time without giving reasons to LUMITOS AG, Ernst-Augustin-Str. 2, 12489 Berlin, Germany or by e-mail at revoke@lumitos.com with effect for the future. In addition, each email contains a link to unsubscribe from the corresponding newsletter.