Organic chemistry research transformed: the convergence of automation and AI reshapes scientific exploration
A generative, self-evolving AI chemistry research assistant
Recently, "National Science Open" magazine published online a review article led by Professor Fanyang Mo (School of Materials Science and Engineering, Peking University) and Professor Yuntian Chen (Eastern Institute of Technology, Ningbo). The research team proposed a significant shift towards automation and artificial intelligence (AI) in organic chemistry over the past decade. Furthermore, they introduced an innovative concept: the development of a generative, self-evolving AI chemistry research assistant.
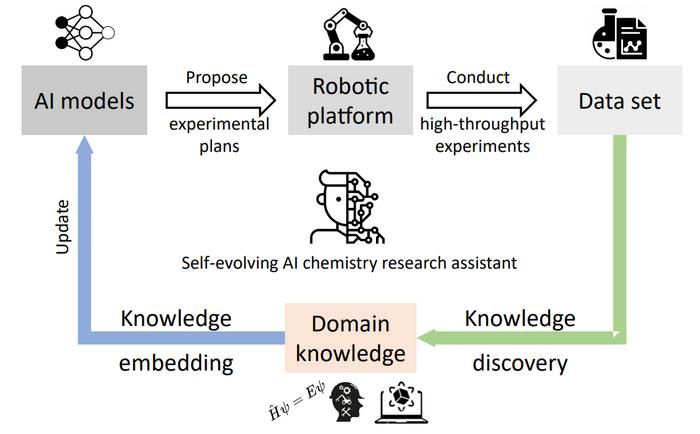
Data produced by advanced robotic systems employing high-throughput methods is processed using artificial intelligence models for decision-making. This dataset undergoes processes of knowledge discovery (green line) and embedding (blue line), enabling the enrichment of the AI's understanding. Subsequently, the AI model undergoes iterative updates, forming a continuous feedback loop that enhances its performance and decision-making capabilities
©Science China Press
The landscape of research in organic chemistry has undergone profound changes. Data, computing power, and sophisticated algorithms constitute the foundational pillars of AI-driven scientific research. In recent years, the rapid advancements in computing technology, coupled with the iterative enhancement of algorithms, have initiated a series of paradigm shifts in the scientific domain. This has led to a complete overhaul of conventional research methodologies. Organic chemistry, inherently predisposed to creating new substances, is uniquely positioned to thrive in this era of intelligent innovation. Scientists globally are now converging in their efforts to explore and harness the capabilities of artificial intelligence in chemistry, thus igniting the 'artificial intelligence chemistry' movement.
The academic realm is currently at the forefront of a research renaissance in this domain. The future holds great promise for the application of knowledge embedding and knowledge discovery techniques in scientific machine learning. This innovative approach is designed to narrow the gap between existing predictive models and automated experimental platforms, thereby facilitating the development of self-evolving AI chemical research assistants. In the field of organic chemistry, the concept of knowledge discovery through scientific machine learning is unlocking new possibilities. At the heart of this discipline is the understanding of reaction mechanisms, which often involve complex networks of intermediates, transition states, and concurrent reactions. Traditional approaches to deciphering these mechanisms have depended on kinetic studies and isotope labeling. However, merging symbolic mathematics with AI is poised to cast new light on these intricate pathways, potentially transforming both the understanding and teaching of organic chemical reactions.
Furthermore, the aspect of knowledge embedding holds significant importance from an organic chemist's perspective. Organic chemistry is replete with heuristic rules, ranging from Markovnikov's rules for electrophilic addition to Baldwin's rules for ring closures. Embedding these established principles into AI models would ensure that their predictions are not solely data-driven but also resonate with the intuitive understanding of chemists. This integration would yield insights that are both deeper and more aligned with the nuanced perspectives of organic chemistry.
Original publication
Other news from the department science
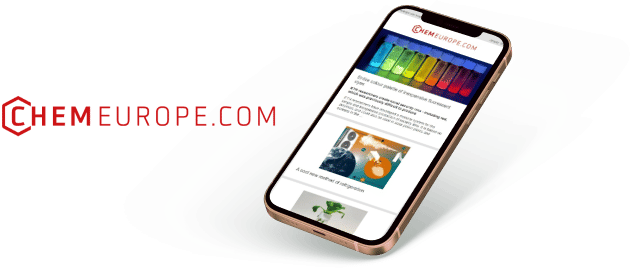
Get the chemical industry in your inbox
By submitting this form you agree that LUMITOS AG will send you the newsletter(s) selected above by email. Your data will not be passed on to third parties. Your data will be stored and processed in accordance with our data protection regulations. LUMITOS may contact you by email for the purpose of advertising or market and opinion surveys. You can revoke your consent at any time without giving reasons to LUMITOS AG, Ernst-Augustin-Str. 2, 12489 Berlin, Germany or by e-mail at revoke@lumitos.com with effect for the future. In addition, each email contains a link to unsubscribe from the corresponding newsletter.