Smart machine maintenance
Avoid unpleasant surprises: New AI system also detects unknown faults
A new maintenance system is helping to make sensors smart. A research team led by Professor Andreas Schütze of Saarland University is combining artificial intelligence with sensors that gather status data on industrial machinery. The system is able to detect damage, wear and error states, and, uniquely, is also able to recognize when previously unknown machine states arise, learning from them and assigning them to their underlying root causes. This approach offers small and medium-sized companies a means of automating their machine maintenance and servicing operations, allowing them to plan more precisely and avoid unpleasant surprises.
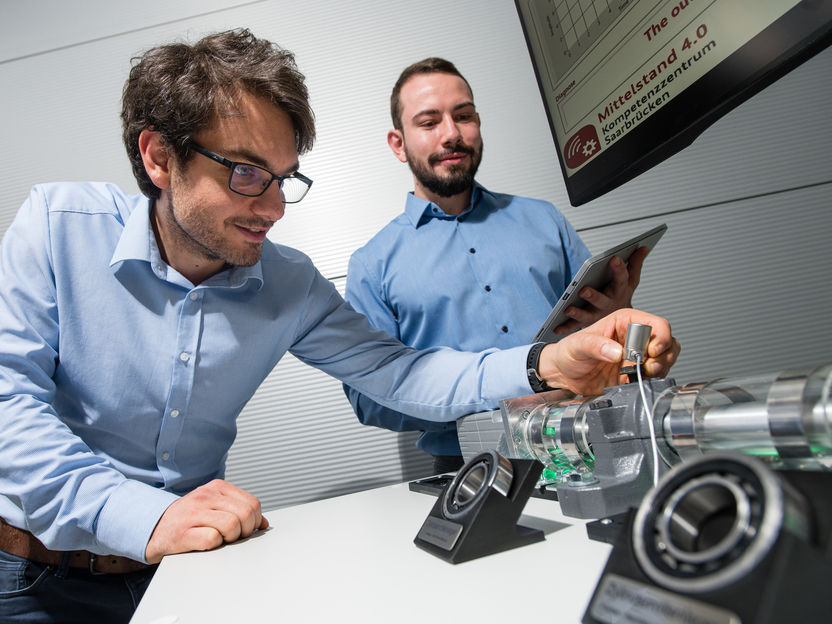
Steffen Klein (l.) and Christopher Schnur, research assistants in the group led by Andreas Schütze, are currently conducting research into the new system.
© Oliver Dietze
Vast numbers of sensors are constantly collecting data from today’s industrial machinery. And there’s a lot that can be learned from these huge data sets. When a machine is operating normally, the way that it vibrates, shakes, hums or heats up is unique to that device. But when machine components start to wear out, these characteristic features undergo subtle changes. Minute temperature fluctuations, slight changes in vibrational behaviour, minor shifts in measurement data can all act as early warning signals that can indicate when a component is beginning to show signs of wear. It is therefore crucial to be able to detect these subtle variations within the sea of data being produced. ‘A single sensor can generate a terabyte of raw data in just a few days,’ explains Professor Andreas Schütze, an expert in measurement and sensor technology at Saarland University. But in addition to detecting these changes, it is equally important to know how to interpret them.
Schütze and his team have been working with partners in industry and academia to develop a system that is able to extract the useful signal data from the vast quantities of data being generated. ‘By independently assigning signal patterns to specific damage, wear or error states, the system is able to make the machine’s status permanently visible,’ says Andreas Schütze. The program continuously compares real-time sensor data with the data associated with normal machine operation and with the typical signal patterns that indicate an incipient malfunction or emerging wear defects. If the system detects a difference between these signal patterns, it will notify the equipment operator and indicate how to respond. The researchers working at Saarland University and at the Center for Mechatronics and Automation Technology (ZeMA) in Saarbrücken have developed a whole suite of hardware and software modules that can be combined to produce a tailor-made monitoring system for a wide variety of industrial machinery and equipment.
The system is even able to detect unknown faults, to learn from them and then to assign these faults to their corresponding root cause. This is something wholly new. Up until now, AI-based monitoring systems were not able to evaluate previously unknown events. ‘Artificial intelligence works by pattern recognition. If something completely new happens and the system doesn’t recognize this novel pattern, it will have effectively reached the limits of its capabilities. We’ve developed our system to a level where it can recognize states that is has not previously encountered and can notify the operator accordingly,’ explains Andreas Schütze. The technical term is ‘novelty detection’. If a novel event begins to appear more frequently and more data on it becomes available, the program is able to assign its cause and the consequences that follow from it.
Over the course of multiple research projects, Schütze’s group filtered out from the vast quantities of measurement data those signal patterns that were associated either with changes in a machine’s behaviour or with machine damage. They then created mathematical models, which included simulations of sensor faults, and used these models to teach their system. The program exploits machine learning techniques to automatically acquire new knowledge and to detect deviations from normal behaviour. ‘The algorithms also incorporate recently acquired data in their analyses. It is therefore possible for the system to detect and to interpret anomalies,’ explains Tizian Schneider, a doctoral student who is currently conducting research into the new system.
The knowledge generated by the system can be linked to other AI functions, such as the automated ordering of spare parts. This makes it easier to plan maintenance operations on large or difficult-to-access plant machinery. The system is also able to transfer information to human maintenance operatives in a clearly understandable form. To ensure that maintenance personnel are able to interpret the numerical data correctly, Schütze’s team has also examined ways of translating the data into useful information for the user. ‘The system breaks down the information into a form that is both relevant and easily understood by the maintenance workers,’ explains Tizian Schneider.
Schütze and his team now want to help small and medium-sized companies become acquainted with the new technology. The researchers run training courses at the ‘Mittelstand 4.0 Competence Centre’, which is located at the ZeMA site in Saarbrücken and which is funded by the Federal Ministry of Economics and Energy. They are currently developing an AI-based assistance system specifically for small and medium-sized companies. ‘The system is particularly attractive for these small and medium-sized enterprises that want to use digitalization to boost their competitiveness.’ explains Andreas Schütze.
Other news from the department science
These products might interest you
Most read news
More news from our other portals
See the theme worlds for related content
Topic world Sensor technology
Sensor technology has revolutionized the chemical industry by providing accurate, timely and reliable data across a wide range of processes. From monitoring critical parameters in production lines to early detection of potential malfunctions or hazards, sensors are the silent sentinels that ensure quality, efficiency and safety.
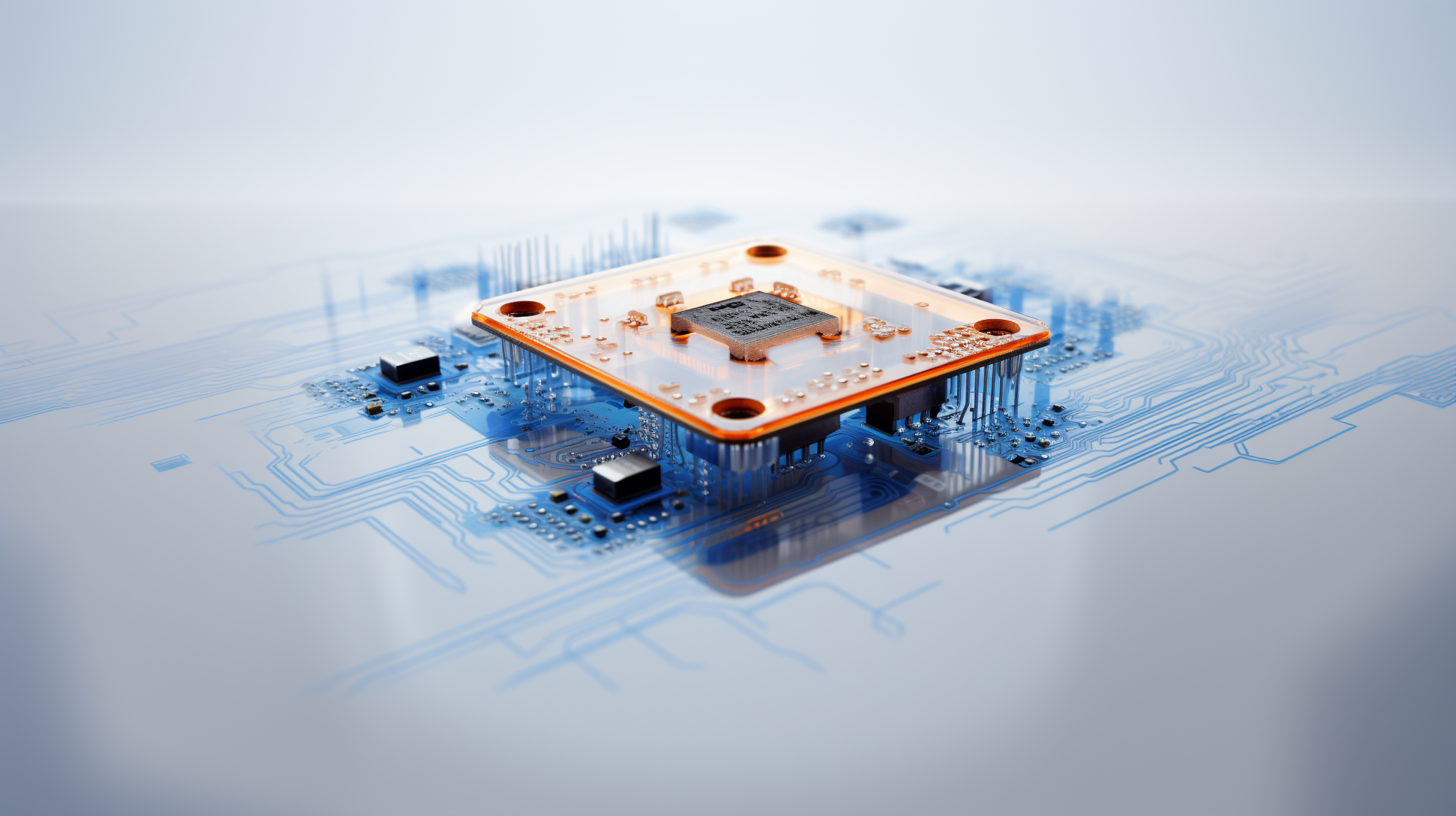
Topic world Sensor technology
Sensor technology has revolutionized the chemical industry by providing accurate, timely and reliable data across a wide range of processes. From monitoring critical parameters in production lines to early detection of potential malfunctions or hazards, sensors are the silent sentinels that ensure quality, efficiency and safety.