Machine learning and AI aiding in the prediction of chemical reaction outcomes
There are few problems now that AI and machine learning cannot help overcome. Researchers from the Yokohama National University use this modern advantage to overcome what conventional methods cannot.
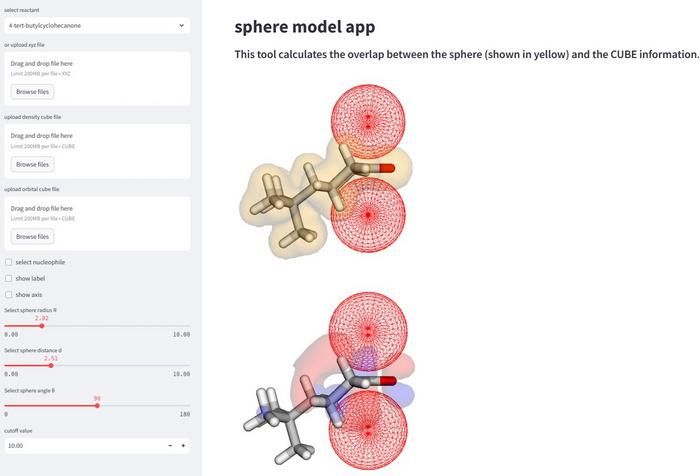
A web application for use by experimental chemists created by us. Uploading a file calculated with commercially available software, and the electronic state can be analyzed. We are working on creating a platform that allows chemists around the world to analyze their own reaction systems.
Yokohama National University
There are many rules to remember when it comes to the interaction of carbon-containing (or organic) molecules. The positioning of groups on the molecule that interact with its environment, the size, shape and position of the molecule, and the molecule it is interacting with. The outcome of a given reaction can be very different depending on these factors and many more, and predicting these outcomes has proven to be quite the challenge in the chemical field. Controlling the outcome is a very necessary component of chemical synthesis, but predictions aren’t always enough. Fortunately, machine learning and artificial intelligence (AI) once again can help push progress forward by predicting the rate or selectivity of a given reaction. Therefore, this technology can be useful in predicting what product can be expected.
Researchers published their findings in the Journal of Chemical Information and Modeling on April 9th.
In organic chemistry, every detail matters. Two common areas that can impact how a molecule interfaces with other molecules are sterics and orbitals. Sterics refer to the arrangement of molecules and steric effects can determine the shape and reactivity of the molecule. This can be due to the size or charge of the molecule or individual atom. Orbitals are a way to explain the most likely placement of the electrons that, in turn, can interact with other molecules or atoms to cause reactions. These factors can drastically change where a nucleophile, or an electron-donating reactant, can attach to the recipient molecule. This is known as the “selectivity” and depending on where the molecule attaches, the results can form different products or yields of the desired product. Researchers are utilizing AI and machine learning as well as the current knowledge of chemical reactions to better explain these aspects of molecular selectivity.
“To determine what information can be used as essential chemical information to be given to AI, it is necessary to combine chemical knowledge with knowledge of AI and machine learning,’ said the corresponding author Hiroaki Gotoh, associate professor at the faculty of engineering, Yokohama National University.
First, the computer had to be fed some information to learn from. Information from literature in computational chemistry and information from previous studies was used to begin the teaching process of the AI. After some manual data entry for the specific molecules being used and setting optimal parameters, data analyses were run based on the predicted outcomes of the test data set. These analyses allow for the AI to learn and predict future selectivities based on information already known. “This method enables a more comprehensive analysis and interpretation of reaction mechanisms via calculation of the parameters of the spherical spaces that mimic approaching nucleophiles,” said Daimon Sakaguchi, first author of the study at the department of chemistry and life science, Yokohama National University.
The study successfully explained the 323 reactions selectivity of eight nucleophiles based on which “face” of the molecule will yield the most desirable amount of product. The selectivity changes based on the sterics of the molecule in addition to its orbital factors. Researchers found that for some molecules the orbital factor is more important in determining facial selectivity, and others are more dependent on the sterics of the molecule when it interacts with its nucleophile.
The combination of predictive tech and machine learning with established knowledge of chemistry can yield better results from the chemical reaction and help chemists synthesize natural products and pharmaceutical chemicals in a more streamlined way.
By streamlining this process with the use of machine learning and artificial intelligence, more experimentation can occur. Ideally, the researchers hope to collaborate with experimental chemists to design reactions that will continue with the development of more predictive technology for chemical reactions.