AI supports analysis of metallic materials
"With our specially developed algorithm, we are three times faster"
Until now, the analysis of crystalline microstructures in metallic materials has been technically challenging and particularly time-consuming. Researchers from the fields of materials engineering and computer science have now developed an algorithm for this purpose and published it in the scientific journal "Scientific Reports": From just a few measurement data of an X-ray structure analysis, it reconstructs the orientation of the crystal structures in the material completely and accurately.
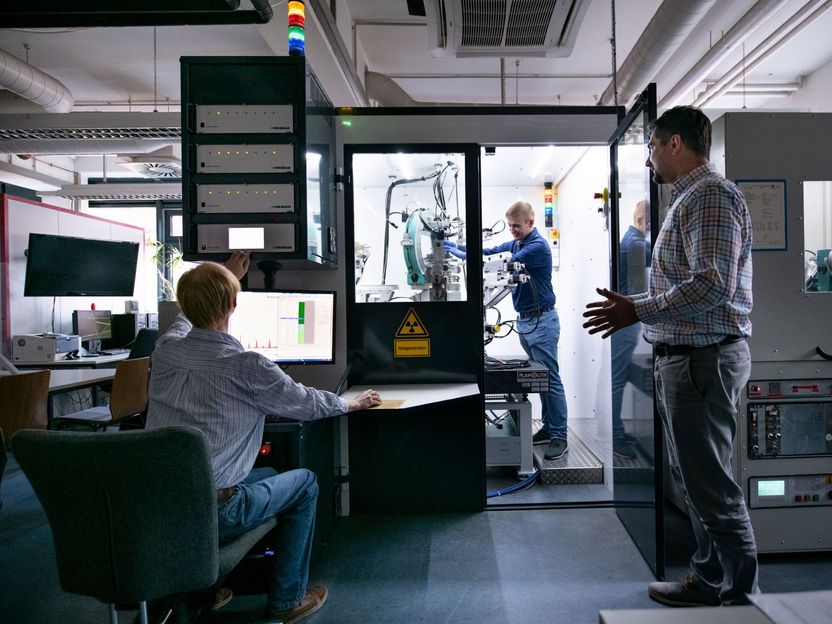
Researchers at the Department of Metallic Materials preparing an X-ray structural analysis.
Paavo Blafield
The inner structure of crystalline individual regions, so-called "grains", is decisive for the properties of metallic materials. Their arrangement has a decisive influence on the strength and deformation behavior of the metals. So-called shape memory alloys, for example, change their shape due to temperature-induced changes in the internal crystal structure. "Creating a suitable microstructure in these special materials is a major technical challenge. Checking this in detail with the help of X-ray analyses is particularly complex," explains Prof. Dr.-Ing. Thomas Niendorf, head of the Metallic Materials department.
For this purpose, researchers often use the methods of X-ray diffraction. In this process, they direct a focused X-ray beam onto the material samples. The beam is deflected at its crystal lattice - in physical terms, diffracted. A detector receives the diffracted X-rays and software displays their intensity in a so-called pole figure. They rotate and tilt the material sample until a pole figure is created from the measurement data. These series of measurements often last for several days. Using the pole figures, the researchers can computationally determine the arrangement and orientation of the crystals in the metal.
"With our specially developed algorithm, we are three times faster," reports David Meier, an information scientist from Helmholtz-Zentrum Berlin and the Department of Intelligent Embedded Systems at the University of Kassel (headed by Prof. Bernhard Sick). "With machine learning, it is trained to create a complete reconstruction of the pole figure from only a small section of real measurement data of a few hours. It differs only minimally from the original." To do this, Meier worked with the materials engineers to create pole figures of random arrangements of grains in the metal using a simulation. Using these simulated images, a customized deep-learning architecture learns to generate the complete pole figure from a section. This "reconstruction network" can reconstruct the remaining areas for a small section of a real measured pole figure. The subsequent comparison of reconstruction and real, complete measurement results of the sample shows: The reconstruction network can analyze the sample with sufficient accuracy for the applied example. But: in order to statistically prove that the developed method works in other real-life scenarios, it needs to be evaluated in follow-up studies with further samples from different materials, says David Meier.
The researchers in the Department of Metallic Materials are enthusiastic about the collaboration with computer science: "We now only need a few hours to analyze the micro-crystal structure and can even reliably reconstruct areas that we would not have been able to reach at all technically with our experimental setup," says Dr.-Ing. Alexander Liehr, head of the X-ray fine structure analysis group. In the future, too, the combination of modern measurement technology and artificial intelligence could support the research and development of high-performance and durable materials.
Original publication
Most read news
Other news from the department science
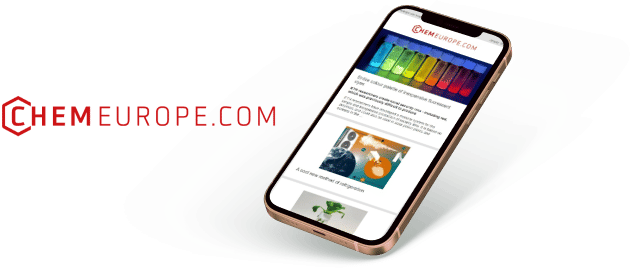
Get the chemical industry in your inbox
By submitting this form you agree that LUMITOS AG will send you the newsletter(s) selected above by email. Your data will not be passed on to third parties. Your data will be stored and processed in accordance with our data protection regulations. LUMITOS may contact you by email for the purpose of advertising or market and opinion surveys. You can revoke your consent at any time without giving reasons to LUMITOS AG, Ernst-Augustin-Str. 2, 12489 Berlin, Germany or by e-mail at revoke@lumitos.com with effect for the future. In addition, each email contains a link to unsubscribe from the corresponding newsletter.
Most read news
More news from our other portals
Last viewed contents
1,3-Butadiene
Pfizer to Acquire BioRexis to Access Novel Technology Platform and Early Stage Diabetes Pipeline
Category:Methyl_indoles
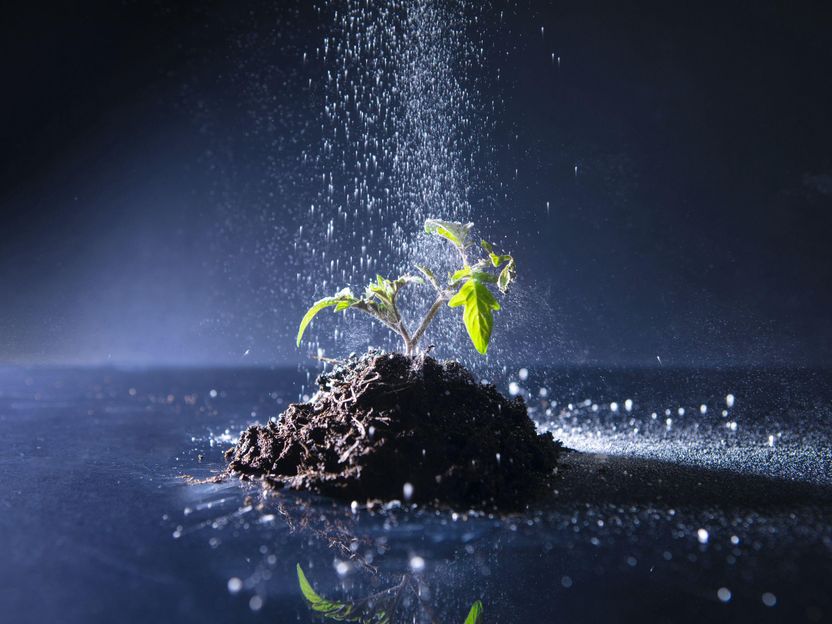
PETRA III shines a light on efficient production technique for a novel ‘green’ fertiliser - Advanced milling technique produces slow-release soil nutrient crystals
Luis_Federico_Leloir
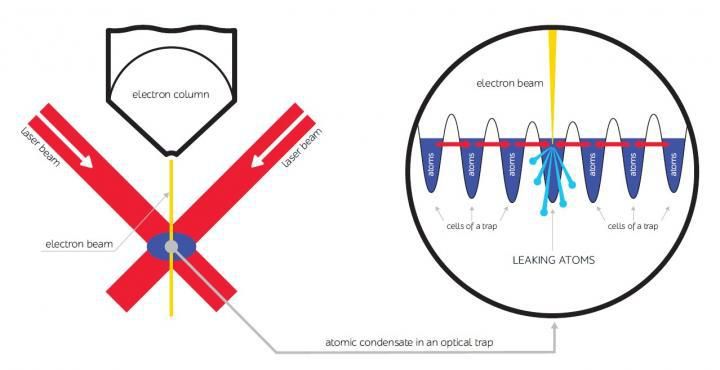
Antilaser for Bose-Einstein condensate
Edgar_Fahs_Smith
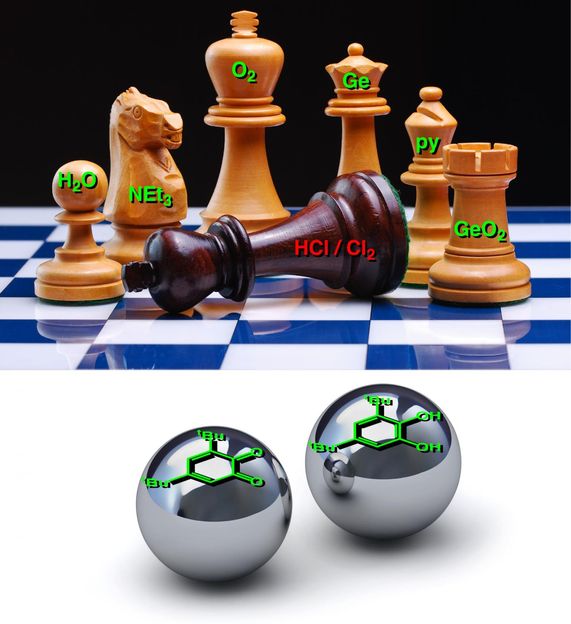