Machine learning model speeds up assessing catalysts for decarbonization technology from months to milliseconds
Scientists create computational model for identifying low-cost catalysts that convert biomass into fuels and useful chemicals with low carbon footprint
Next time you drive past farms or prairies and ponds on a rural road, look around. They are a rich source of biomass. That includes corn, soybeans, sugar cane, switchgrass, algae and other plant matter. These carbon-rich materials can be converted to liquid fuels and chemicals with many possible applications. There is enough biomass in the United States, for example, to produce renewable jet fuel for all air travel.
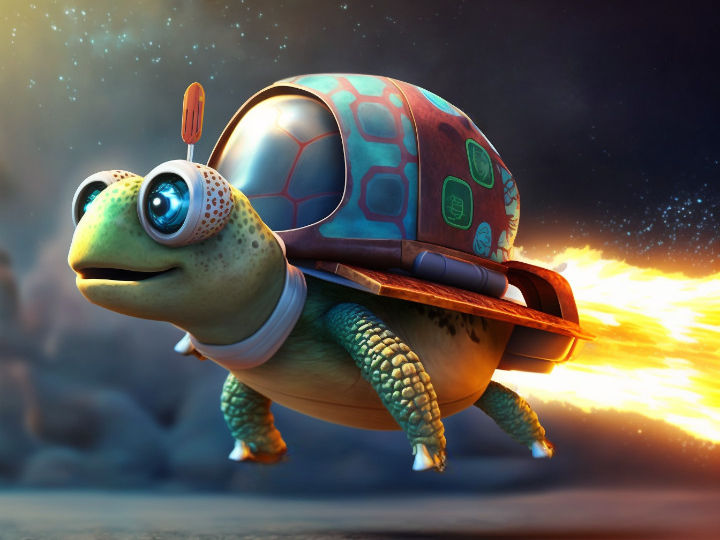
From months to milliseconds: Slow becomes fast (symbolic image)
Computer-generated image
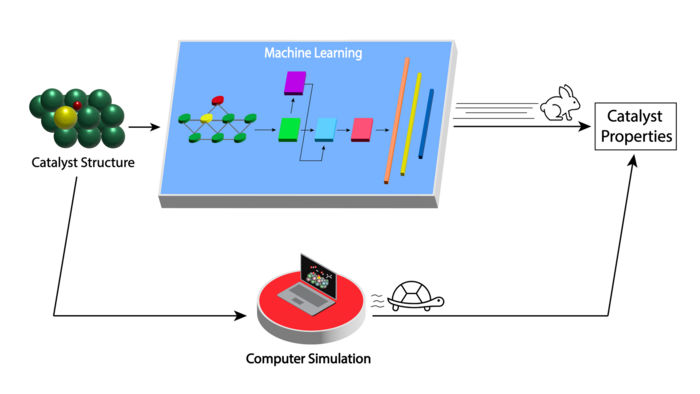
The newly developed machine learning model greatly speeds up assessing the properties of molybdenum carbide catalysts for biomass conversion to useful products (top path) compared with current computer simulation methods (bottom path).
Image by Argonne National Laboratory
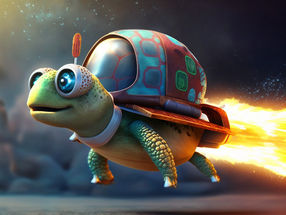
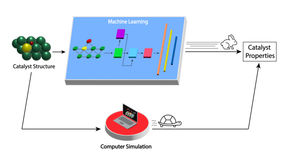
A major stumbling block at present is lack of effective, low-cost catalysts needed to transform biomass into biofuel or other useful products. Researchers at the U.S. Department of Energy’s (DOE) Argonne National Laboratory report developing an artificial intelligence-based model to speed up the process for engineering a low-cost catalyst based on molybdenum carbide.
“Biomass is an organic material, meaning it is full of carbon,” said Rajeev Assary, group leader in Argonne’s Materials Science Division (MSD). “The ultimate goal is to cheaply transform that carbon into useful products for society, in this case, biofuel and chemicals such as biodegradable plastic. These products avoid the need for fossil fuel.”
At present, scientists can produce a petroleum-like product called pyrolysis oil by treating raw biomass at high temperatures. But the resulting product has a very high oxygen content. That oxygen is undesirable and therefore removed by a reaction enabled by use of a molybdenum carbide catalyst. But a major problem has been that the surface of this catalyst absorbs oxygen atoms, which accumulate on the surface and degrade catalyst performance.
One proposed solution is to add to the molybdenum carbide a small amount of a new element, such as nickel or zinc. This dopant element weakens the bonding of the oxygen atoms on the catalyst surface, preventing catalyst poisoning.
“The problem is to find the right combination of dopant and surface structure,” said Hieu Doan, an assistant scientist in MSD. “Molybdenum carbide has a very complicated structure. We thus called upon supercomputing combined with theoretical calculation to simulate the behavior for not only the surface atoms binding with oxygen but also atoms nearby.”
Using simulations carried out on Argonne’s Theta supercomputer, the team created a database of 20,000 structures for the binding energies of oxygen to doped molybdenum carbide. Their simulations took into account several dozen dopant elements and over a hundred possible positions for each dopant on the catalyst surface. Theta is part of the Argonne Leadership Computing Facility, a DOE Office of Science user facility.
Then, they used this database to train a deep learning model. Deep learning is a form of machine learning in which the computer learns to solve problems by first analyzing a large sample set of data. “Instead of being limited to evaluating a few thousand catalyst structures over months with conventional computational methods, with our deep learning model we can now do accurate and inexpensive calculations for tens of thousands of structures in milliseconds,” said Doan. “It is materials screening on steroids.”
The team sent the results from their atomic-scale simulations and deep learning model to the Chemical Catalysis for Bioenergy Consortium. They will be conducting experiments to evaluate a small set of candidate catalysts.
“In the near future, we hope to handle over a million structures and different binding atoms, such as hydrogen,” noted Assary. “We also want to apply this same computational approach to catalysts for other decarbonization technologies, such as the conversion of water into clean hydrogen fuel.”