How to play Catalysis Tetris?
The way molecules involved in a chemical reaction bond to the surface of a catalyst is key to improving catalyst performance. Researchers at the Fritz Haber Institute and Denmark's Aarhus University have now developed a machine learning algorithm that can predict this property for complex molecules that can attach to the surface in different motifs.
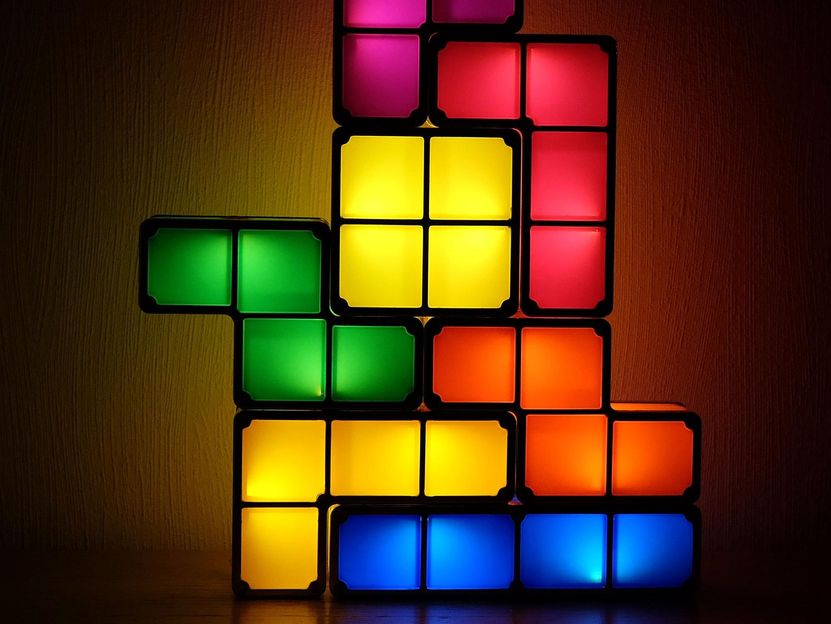
Bild von Tobias Kozlowski auf Pixabay
Do you like to play Tetris? Especially in the higher levels, when you have to push the intricately shaped pieces to the best place very quickly? In a way, scientists in computational catalysis research often have to master a similar task. One of the core variables they regularly calculate using sophisticated quantum mechanical simulation software is how strongly a molecule binds to the surface of a potential catalyst material. The relevance of this information was discovered about a hundred years ago by Nobel Prize winner Paul Sabatier: If the bond is too weak, the molecule is not sufficiently activated to be efficiently converted in the catalytic reaction. If the bond is too strong, it no longer participates in the reaction, but simply blocks the catalyst surface.
Quantum mechanical calculations can provide this important information very accurately, and this is increasingly being used to make an initial assessment of the suitability of a new catalyst material before the material is even labor-intensively synthesized for real in the laboratory. Unfortunately, the calculations require supercomputers, and just like in Tetris, there are different positions on the surface that the molecule could attach to. By trying out all the positions, each with its own calculation, the scientists determine where the molecule fits best. Larger, complicated molecules can also bind to positions in different ways and orientations - again, just like a complicated shaped piece in Tetris. Considering that dozens of molecules are involved, especially in important catalytic reactions such as the Fischer-Tropsch reaction to produce synthetic fuels, it simply takes too long to perform the enormous total number of calculations required. Game over.
Experienced Tetris players develop a sense of where best to push the pieces. "Machine learning algorithms work the same way," explains Wenbin Xu, a doctoral student at the Fritz Haber Institute. After being trained with the results of previous calculations for similar molecules and catalyst surfaces, these algorithms can make reliable predictions about binding without the need for more supercomputer time and much, much faster. No wonder the development of appropriate algorithms is a hot topic. So far, however, the algorithms have not been able to deal properly with more complicated molecules. They could only predict the binding of small molecules that could bind to the surface in only one obvious orientation - like a simple square Tetris piece. "The missing information for the algorithms was the linkage within the molecule: which atom binds to which...," describes Prof. Mie Andersen of Aarhus University. Using mathematical graph theory, the research team has now found a way to incorporate this information appropriately. Their new machine learning algorithm, published in Nature Computational Science, already provides accurate binding information for larger molecules centrally involved in Fischer-Tropsch and other fuel-generating reactions. Catalysis researchers now have a powerful Tetris player on their side.
Note: This article has been translated using a computer system without human intervention. LUMITOS offers these automatic translations to present a wider range of current news. Since this article has been translated with automatic translation, it is possible that it contains errors in vocabulary, syntax or grammar. The original article in German can be found here.
Original publication
"Predicting binding motifs of complex adsorbates using machine learning with a physics-inspired graph representation."; Nature Computational Science 2, 443 (2022)
Most read news
Original publication
"Predicting binding motifs of complex adsorbates using machine learning with a physics-inspired graph representation."; Nature Computational Science 2, 443 (2022)
Organizations
Other news from the department science
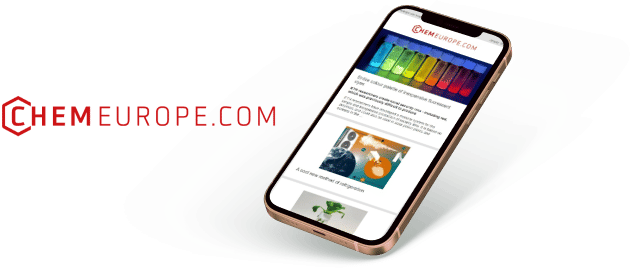
Get the chemical industry in your inbox
By submitting this form you agree that LUMITOS AG will send you the newsletter(s) selected above by email. Your data will not be passed on to third parties. Your data will be stored and processed in accordance with our data protection regulations. LUMITOS may contact you by email for the purpose of advertising or market and opinion surveys. You can revoke your consent at any time without giving reasons to LUMITOS AG, Ernst-Augustin-Str. 2, 12489 Berlin, Germany or by e-mail at revoke@lumitos.com with effect for the future. In addition, each email contains a link to unsubscribe from the corresponding newsletter.