Toward new solar cells with active learning
To deal with the myriad of possibilities for candidate molecules, the machine decides for itself which data it needs
Scientists from the Theory Department of the Fritz-Haber Institute in Berlin and Technical University of Munich use machine learning to discover suitable molecular materials. To deal with the myriad of possibilities for candidate molecules, the machine decides for itself which data it needs.
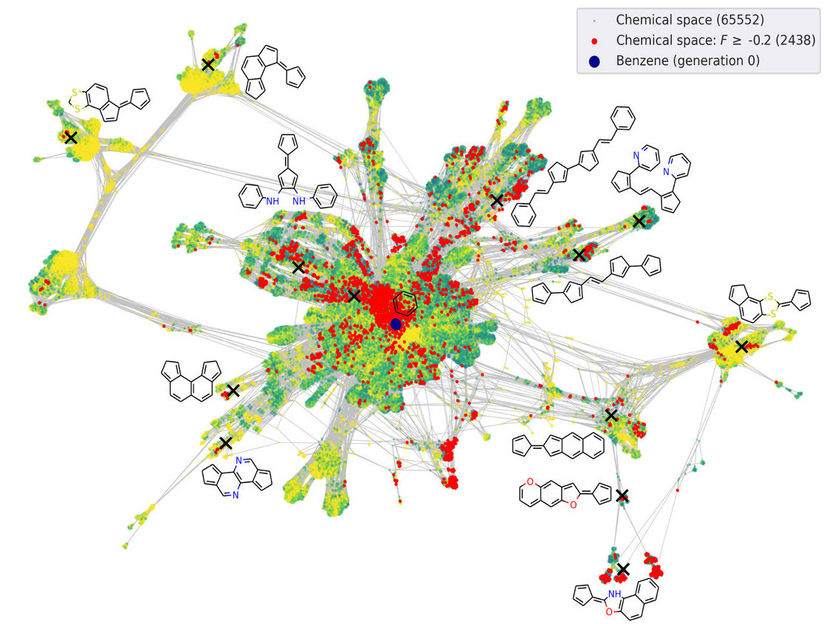
Visualization of the chemical space explored so far.
© Kunkel/FHI
How can I prepare myself for something I do not yet know? Scientists from the Fritz Haber Institute in Berlin and from the Technical University of Munich have addressed this almost philosophical question in the context of machine learning. Learning is no more than drawing on prior experience. In order to deal with a new situation, one needs to have dealt with roughly similar situations before. In machine learning, this correspondingly means that a learning algorithm needs to have been exposed to roughly similar data. But what can we do if there is a nearly infinite amount of possibilities so that it is simply impossible to generate data that covers all situations?
This problem comes up a lot when dealing with an endless number of possible candidate molecules. Organic semiconductors enable important future technologies such as portable solar cells or rollable displays. For such applications, improved organic molecules – which make up these materials – need to be discovered. Tasks of this nature are increasingly using methods of machine learning, while training on data from computer simulations or experiments. The number of potentially possible small organic molecules is, however, estimated to be on the order of 1033. This overwhelming number of possibilities makes it practically impossible to generate enough data to reflect such a large material diversity. In addition, many of those molecules are not even suitable for organic semiconductors. One is essentially looking for the proverbial needle in a haystack.
In their work published recently in Nature Communications the team around Prof. Karsten Reuter, Director of the Theory Department at the Fritz-Haber-Institute, addressed this problem using so-called active learning. Instead of learning from existing data, the machine learning algorithm iteratively decides for itself which data it actually needs to learn about the problem. The scientists first carry out simulations on a few smaller molecules, and obtain data related to the molecules’ electrical conductivity – a measure of their usefulness when looking at possible solar cell materials. Based on this data, the algorithm decides if small modifications to these molecules could already lead to useful properties or whether it is uncertain due to a lack of similar data. In both cases, it automatically requests new simulations, improves itself through the newly generated data, considers new molecules, and goes on to repeat this procedure. In their work, the scientists show how new and promising molecules can efficiently be identified this way, while the algorithm continues its exploration into the vast molecular space, even now, at this very moment. Every week new molecules are being proposed that could usher in the next generation of solar cells and the algorithm just keeps getting better and better.
Original publication
Other news from the department science
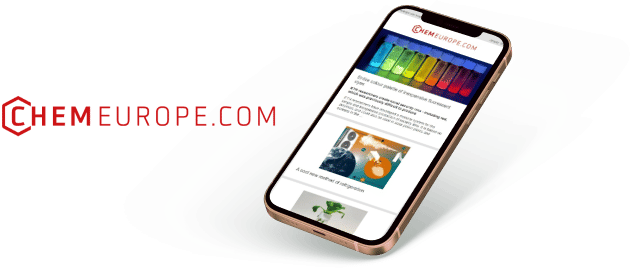
Get the chemical industry in your inbox
By submitting this form you agree that LUMITOS AG will send you the newsletter(s) selected above by email. Your data will not be passed on to third parties. Your data will be stored and processed in accordance with our data protection regulations. LUMITOS may contact you by email for the purpose of advertising or market and opinion surveys. You can revoke your consent at any time without giving reasons to LUMITOS AG, Ernst-Augustin-Str. 2, 12489 Berlin, Germany or by e-mail at revoke@lumitos.com with effect for the future. In addition, each email contains a link to unsubscribe from the corresponding newsletter.