Computers excel in chemistry class
Machine learning models can rapidly and accurately estimate key chemical parameters related to molecular reactivity
Creating Computers that can teach themselves how chemical structure dictates the fundamental properties of molecules and then using that knowledge to predict the properties of novel molecules could help to design cleaner energy and industrial systems.
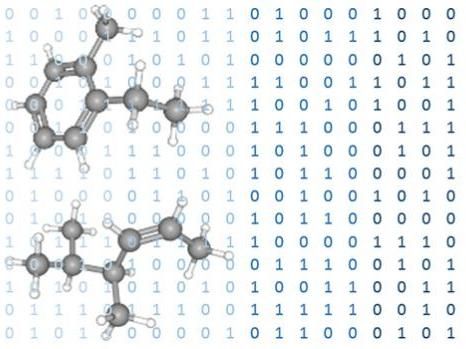
KAUST researchers are converging machine learning with generating thermodynamic data.
© 2020 KAUST
KAUST researchers have developed a machine learning model that can analyze the structure of hydrocarbon molecules and accurately predict a property called enthalpy of formation. When it comes to estimating this property, the model already makes better predictions than conventional approaches, and its accuracy will only improve as more data is collected for the model to learn from.
"Data on molecular properties, such as enthalpy of formation, are essential for engineers modeling the kinetic mechanisms, or energy flows, of chemical reactions," says Kiran Yalamanchi, a Ph.D. student in the research group of Mani Sarathy, who led the research. "Kinetic mechanisms for hydrocarbon fuels are important for the development and optimization of engine designs and chemical reactors," Yalamanchi says.
Generating the large sets of thermodynamics data required for kinetic mechanism modeling typically uses an approach called group additivity, which has limited accuracy. "Group additivity was developed in the mid-20th century, and the field of data science has advanced a lot in the last few decades," Yalamanchi says.
So Yalamanchi and Sarathy approached KAUST computer scientist, Xin Gao, to apply machine learning to the problem. "Our initial study gave very promising results," Yalamanchi says. "This potential helped us to push toward converging machine learning with generating thermodynamic data."
Machine learning offers a way to take enthalpy of formation data--measured experimentally, or calculated for a small number of molecules using highly accurate but slow quantum chemistry computations--and then extrapolate to a much broader range of molecules.
The machine learning program analyzed a "training" dataset of molecule structures and their enthalpies of formation. It then used the patterns it detected to predict the enthalpy of formation of molecules it had not seen before.
Machine learning proved to be much more accurate than the traditional group additivity approach. "We got better estimates of enthalpy of formation of chemical species using machine learning methods compared to traditional methods," Yalamanchi says.
For example, although traditional group additivity can make relatively good predictions for simple molecules with linear structures, its accuracy decreases with more complex molecules, such as those that incorporate carbon rings in their structure. "The improvement we saw in estimates of enthalpy of formation, compared with traditional group additivity, was even more significant in the case of cyclic species," Yalamanchi adds.
"The results suggest that machine learning will become an increasingly important tool in the field," Sarathy says. "The ability to accurately predict important thermodynamic properties from molecular descriptors is an important step toward developing fully automated algorithms for predicting more complex chemical phenomenon," he adds.
The team is now running high accuracy quantum chemistry calculations to expand the machine learning models' training dataset. "In this way, we are developing a hybrid first-principles artificial intelligence framework for more accurate predictions of many physical-chemical properties," says Sarathy.